About MD.ai
MD.ai was founded by Harvard/Duke/Columbia-trained doctors to accelerate the application of AI in medicine, with a particular focus on medical imaging and software tools. With our data annotation platform, we help doctors, scientists, and engineers build high-quality datasets to train and validate AI models. With our AI-powered clinical reporting software, we give radiologists superpowers.
We care deeply that our software and platform have the potential to not only boost the efficiency and productivity of healthcare providers, but also improve patient care and outcomes as well. This commitment drives our mission and propels us forward.
Our software is used within top academic medical institutions as well as large pharmaceutical and healthcare companies.
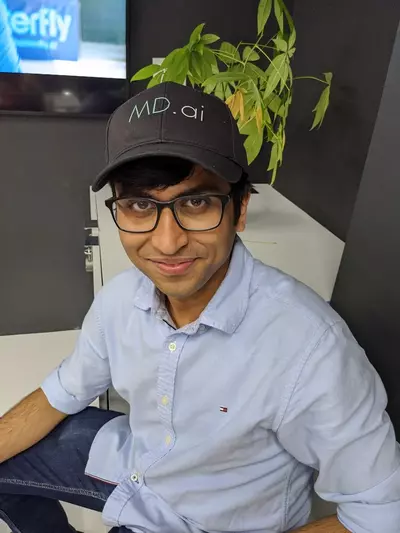
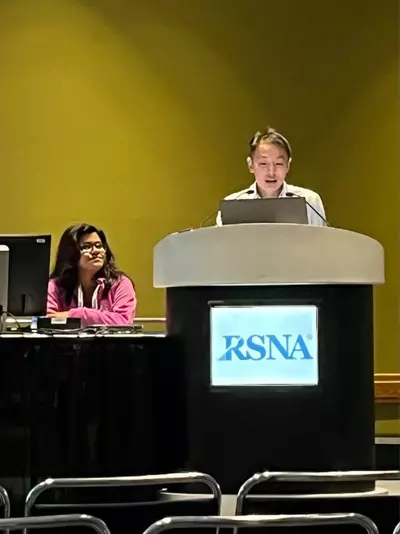
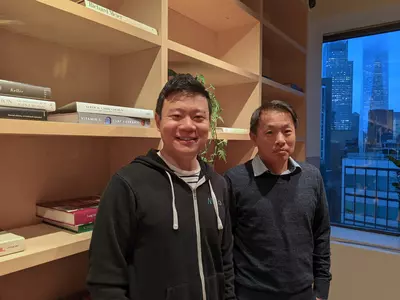
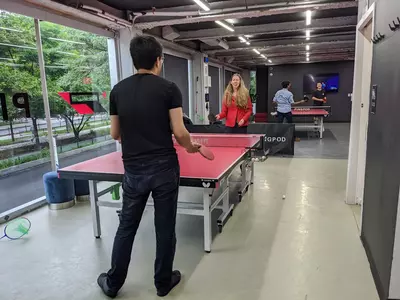
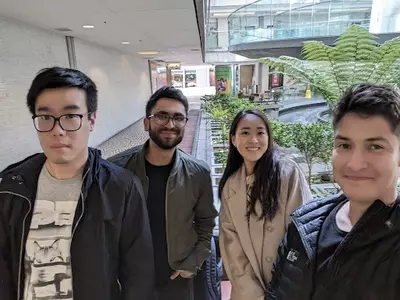
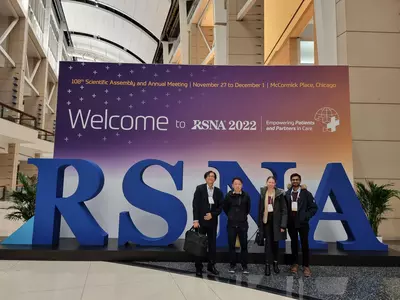
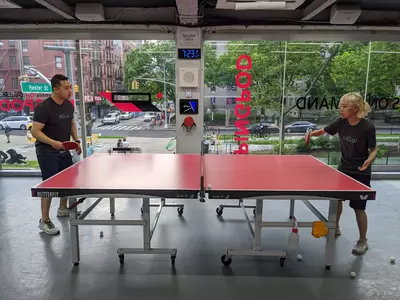
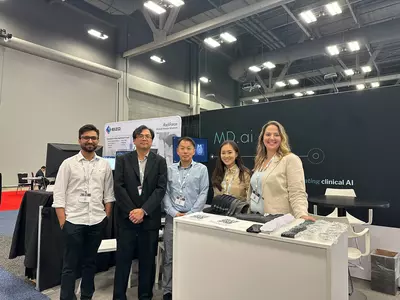
Recent Projects
Showcase of selected public projects and datasets
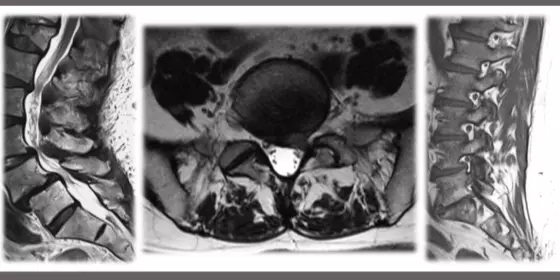
RSNA 2024 Lumbar Spine Degenerative Classification
Classify lumbar spine degenerative conditions.
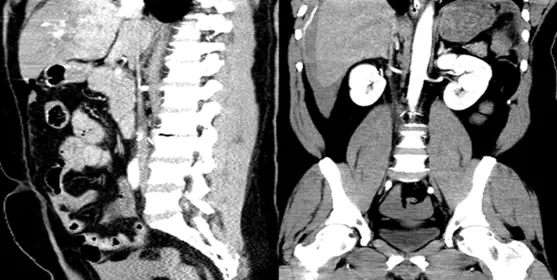
RSNA 2023 Abdominal Trauma Detection
Detect and classify traumatic abdominal injuries.
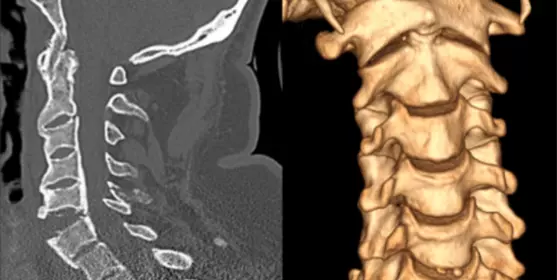
RSNA 2022 Cervical Spine Fracture Detection
Identify cervical fractures from scans.
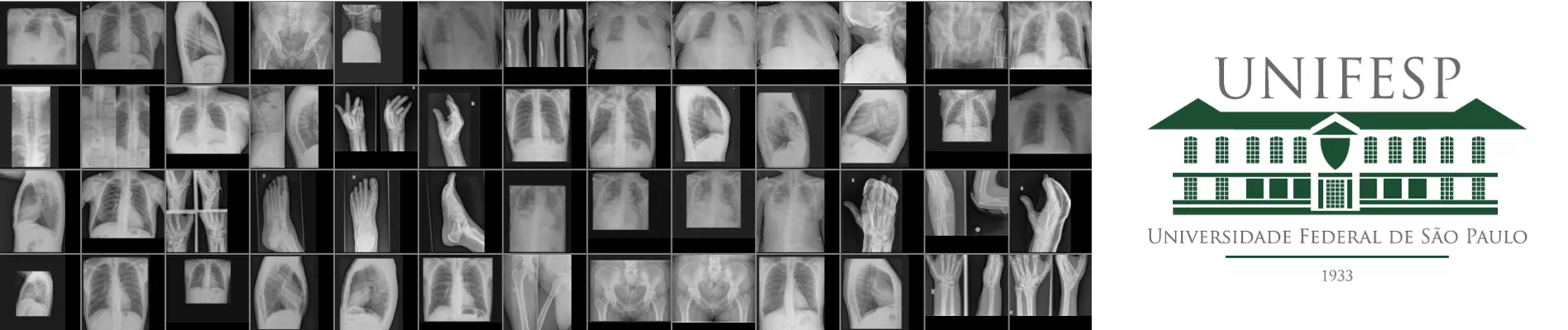
UNIFESP X-ray Body Part Classifier Competition
Can you build an algorithm to correctly classify body parts in X-rays?
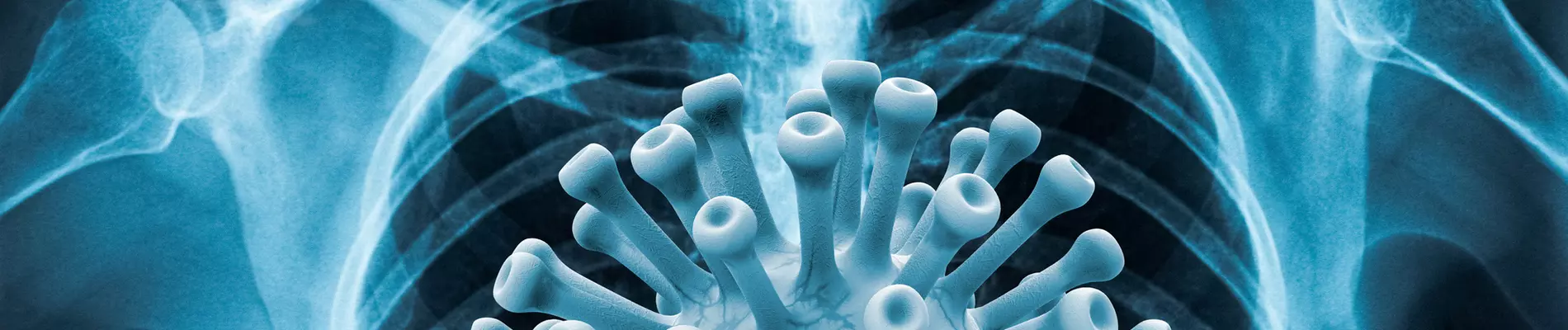
SIIM-FISABIO-RSNA COVID-19 Detection
Identify and localize COVID-19 abnormalities on chest radiographs.
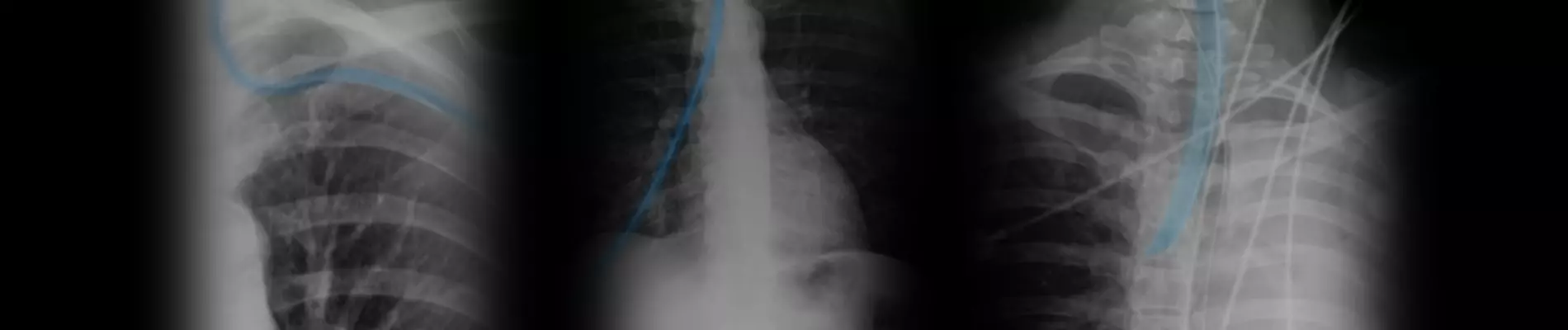
RANZCR CLiP - Catheter and Line Position Challenge
Classify the presence and correct placement of tubes on chest x-rays to save lives.
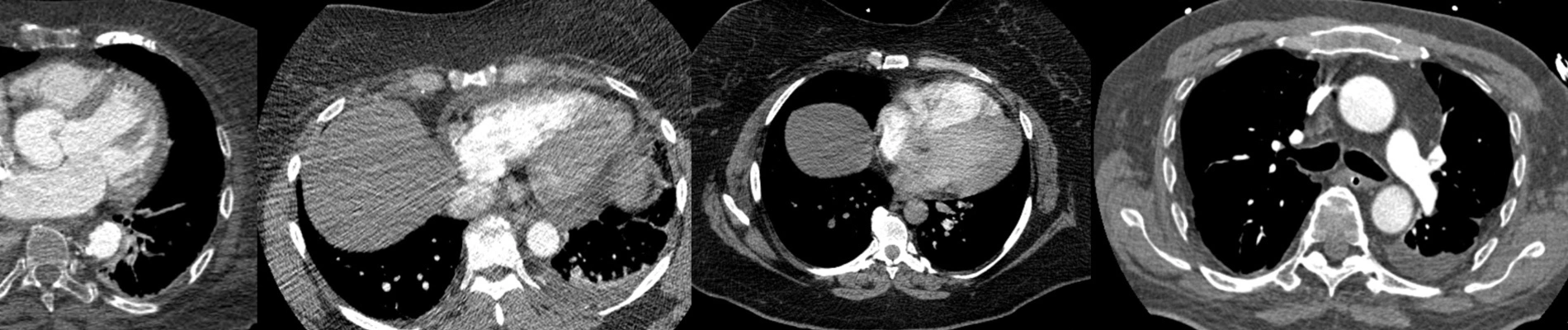
RSNA STR Pulmonary Embolism Detection
The 2020 RSNA Pulmonary Embolism Detection Challenge invited researchers to develop machine-learning algorithms to detect and characterize instances of pulmonary embolism (PE) on chest CT studies. The competition, conducted in collaboration with the Society of Thoracic Radiology (STR), involved creating the largest publicly available annotated PE dataset, comprised of more than 12,000 CT studies. Imaging data was contributed by five international research centers and labeled with detailed clinical annotations by a group of more than 80 expert thoracic radiologists.
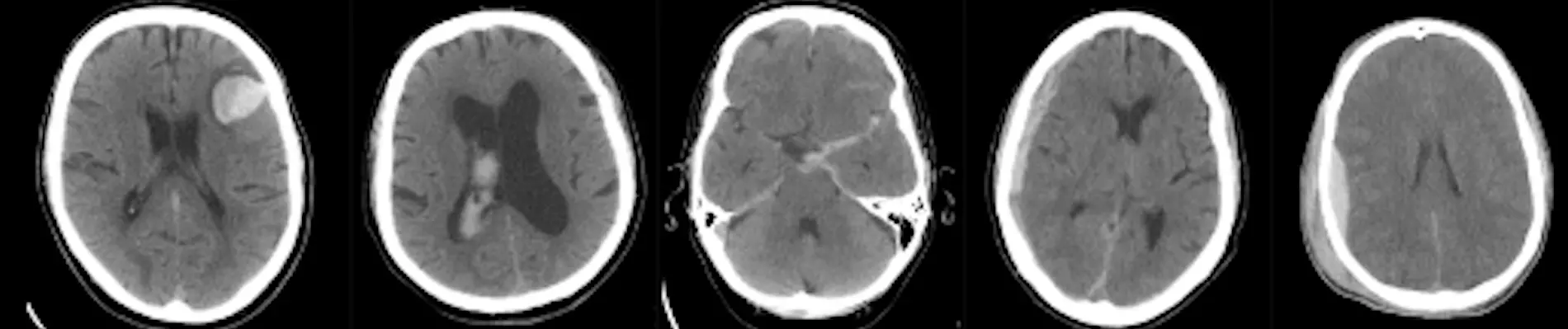
RSNA Intracranial Hemorrhage Detection
The dataset for this Kaggle challenge was created on the MD.ai platform in collaboration with the Radiological Society of North America (RSNA) and the American Society of Neuroradiology (ASNR), with data contributions from Stanford University, St. Michael's Hospital, Thomas Jefferson University, and Universidade Federal de São Paulo.
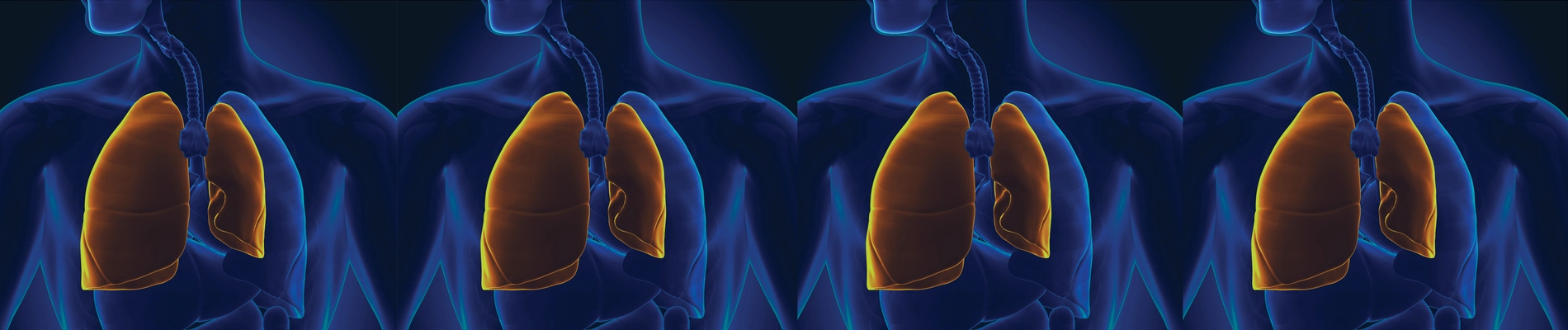
SIIM-ACR Pneumothorax Segmentation
The dataset for this Kaggle challenge was created on the MD.ai platform in collaboration with the Society for Imaging Informatics in Medicine (SIIM) and the Society of Thoracic Radiology (STR).
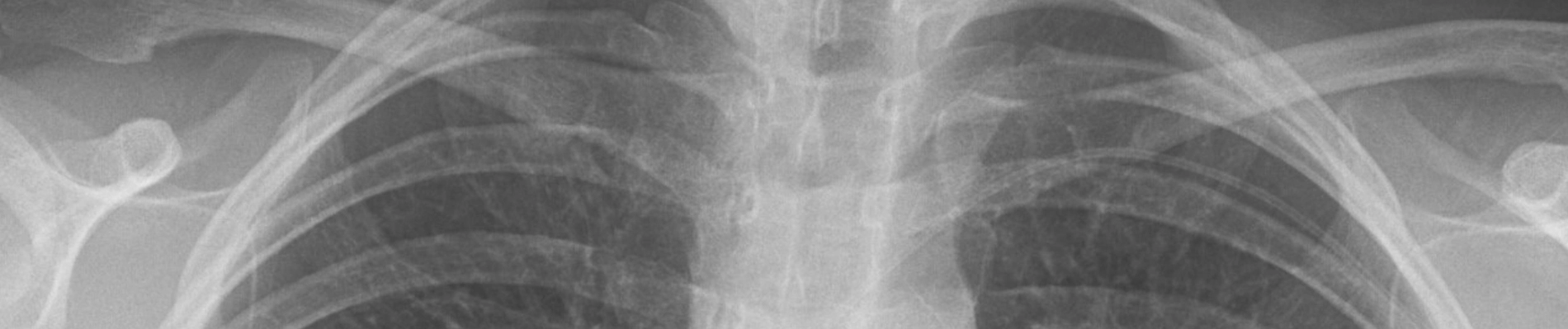
RSNA Pneumonia Detection Challenge
The dataset for this Kaggle challenge was created on the MD.ai platform in collaboration with the Radiological Society of North America (RSNA) and the Society of Thoracic Radiology (STR).
White Papers & Publications
Selected white papers and publications from our team
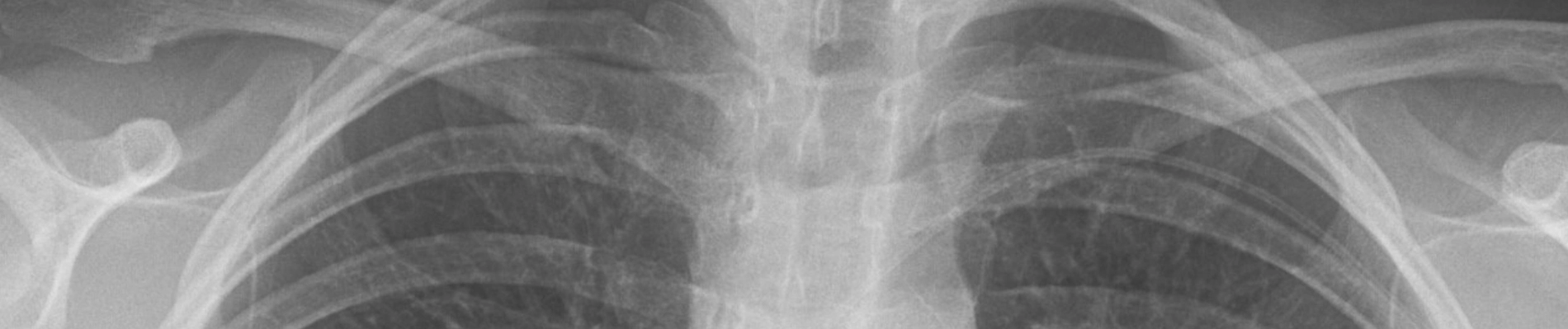
Evaluating GPT-4V (GPT-4 with Vision) on Detection of Radiologic Findings on Chest Radiographs
This study examined the application of GPT-4 with vision (GPT-4V), a multimodal large language model with visual recognition, in detecting radiologic findings from a set of 100 chest radiographs and suggests that GPT-4V is currently not ready for real-world diagnostic usage in interpreting chest radiographs.
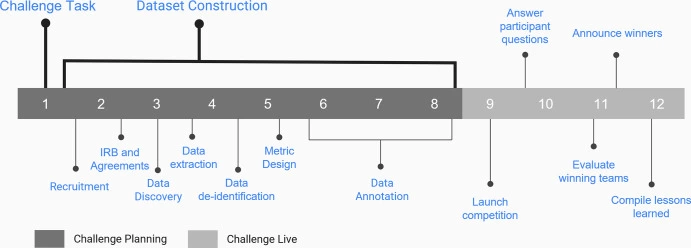
Lessons Learned in Building Expertly Annotated Multi-Institution Datasets and Hosting the RSNA AI Challenges
Organizing AI competitions for medical imaging, such as those by the RSNA, involves complex dataset creation and curation, addressing patient privacy, and ensuring data quality and consistency. These competitions foster global collaboration, advance research, and have the potential to transform healthcare by improving diagnostic accuracy and patient outcomes.
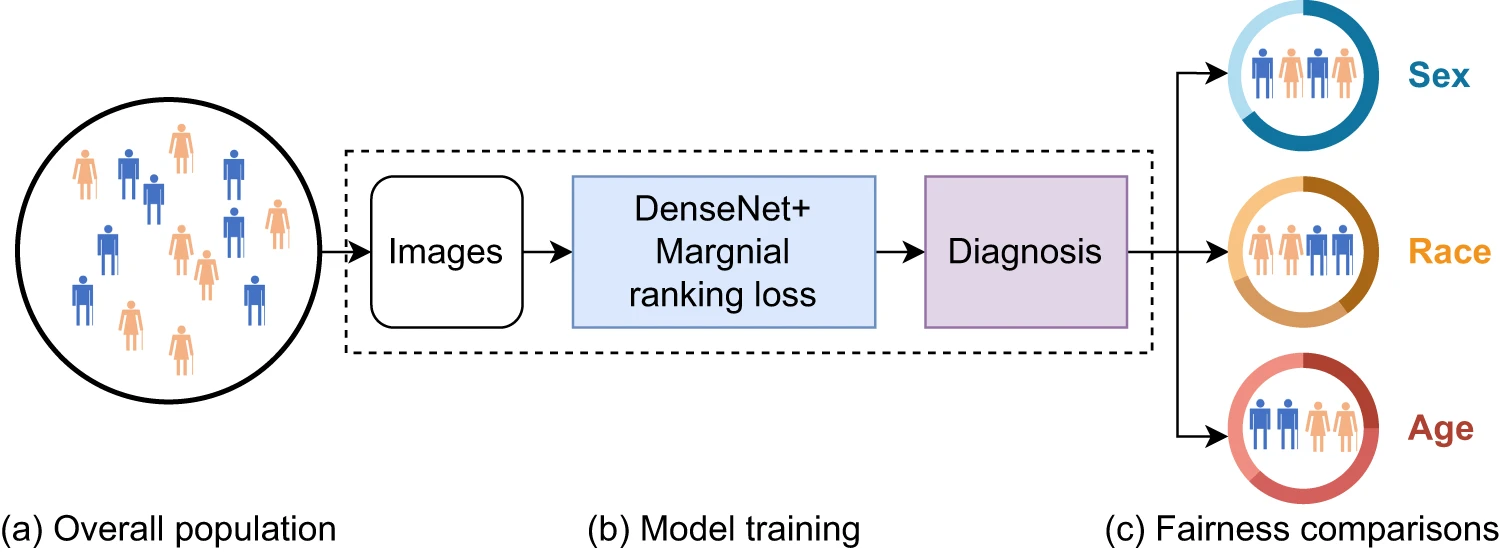
Improving model fairness in image-based computer-aided diagnosis
Deep learning models for medical image classification can match or exceed clinician performance but may amplify human biases, leading to inaccuracies. This study introduces an algorithm to enhance model fairness using marginal pairwise equal opportunity, showing a 35% reduction in fairness disparity without significantly impacting overall performance across four large-scale cohorts.
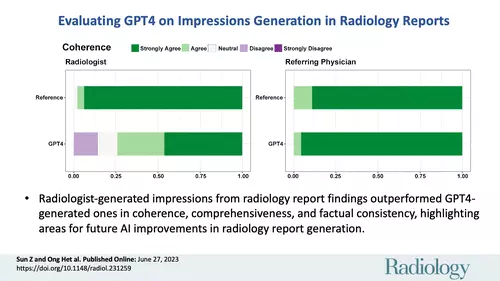
Evaluating GPT-4 on Impressions Generation in Radiology Reports
In this study, we systematically examined the capabilities and limitations of GPT-4 in performing zero-shot generation of Impressions from radiology report Findings. We evaluated the performance of GPT-4 against radiologist-generated Impressions along several predefined dimensions in our previous works—-coherence, comprehensiveness, factual consistency, and harmfulness—-to provide new insights into the feasibility of using large language models in radiology report generation and summarization.
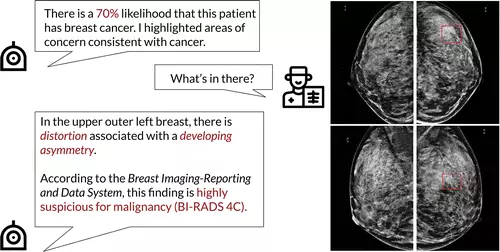
ChatGPT and Other Large Language Models Are Double-edged Swords
This editorial provides several use cases to illustrate the potential role of these tools in the clinical setting and their potential effects on medical journalism.
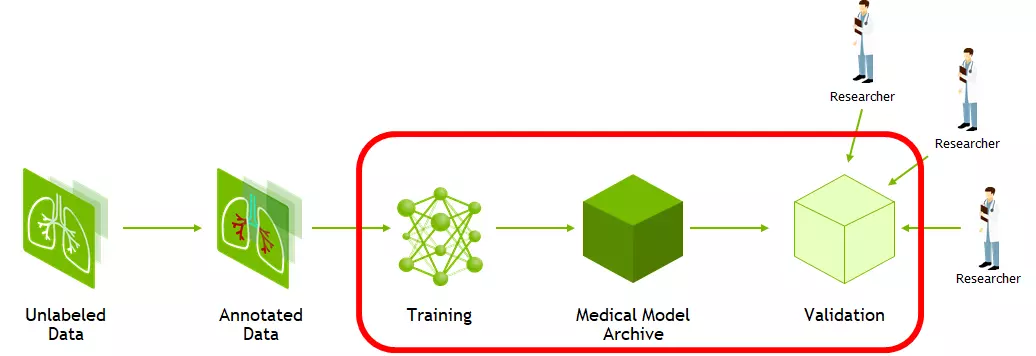
Validating AI Models Collaboratively with NVIDIA Clara Imaging and MD.ai
In this post, we highlighted key components of each platform and the steps necessary to quickly deploy a medical imaging model built with NVIDIA Clara on MD.ai.
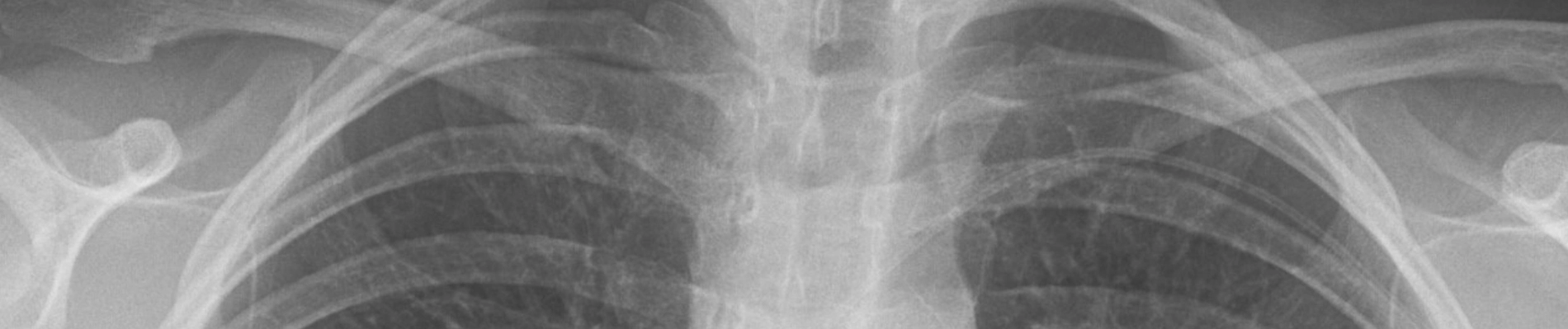
White Paper: RSNA Pneumonia Detection Challenge
Description of the pneumonia crowdsourcing project and adjudication process.
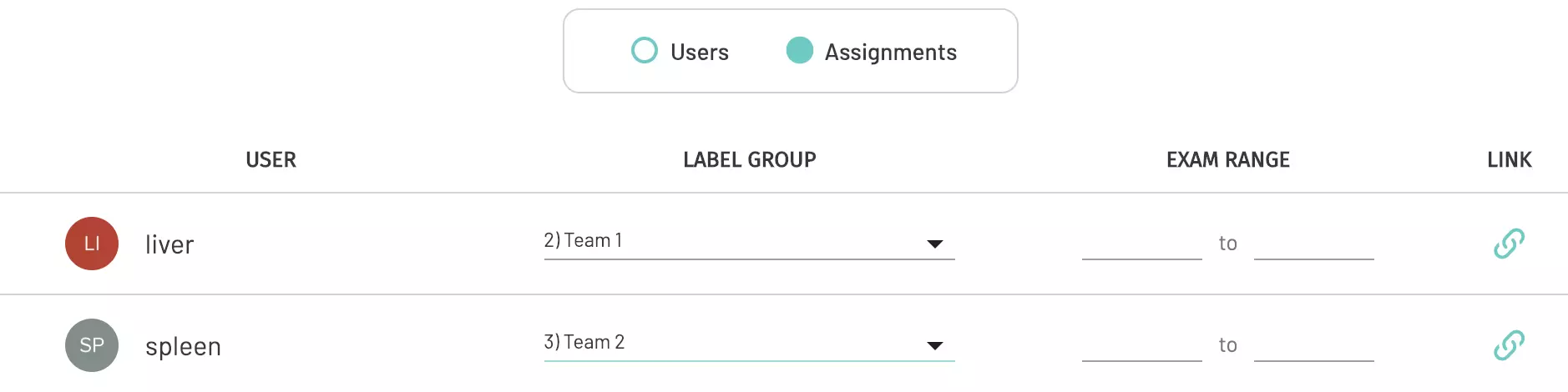
White Paper: Crowdsourcing Annotations
Description of crowdsourced annotations for the RSNA Pneumonia Challenge.
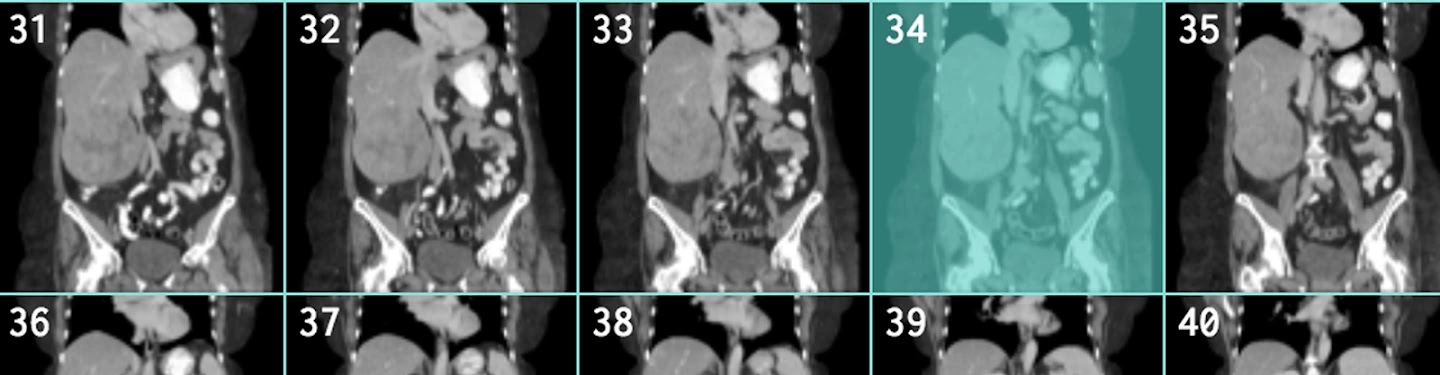
Google Cloud Platform Case Study
MD.ai leverages Google Cloud and Cloud Healthcare API to create annotated datasets and build algorithms for machine learning to bring better insights to medical providers.